In the current rapidly-evolving digital landscape, organizations are keen to establish authentic relationships with their customers. A highly effective strategy for achieving this is through machine learning in email personalization. By employing advanced algorithms and analyzing data, companies can tailor their email marketing strategies, leading to improved engagement levels and heightened customer satisfaction. This article will delve into the nuances of email personalization and investigate how machine learning is poised to revolutionize this approach.
Exploring Email Personalization
Email personalization goes far beyond merely adding a recipient’s name to the subject line. It entails providing content that strikes a chord with individual preferences, behaviors, and requirements. As the digital market grows more saturated, personalized emails have become an essential strategy for marketers to differentiate themselves and effectively engage their audience.
There are various ways to personalize, such as offering relevant product suggestions, crafting messages based on past interactions, and delivering targeted promotions. By tapping into data-driven insights, businesses can offer a more tailored experience for each subscriber, ultimately boosting conversions and fostering brand loyalty.
The Importance of Email Personalization
The significance of email personalization cannot be understated. Research has shown that personalized emails yield higher open and click-through rates compared to generic messages. When recipients feel that an email is tailored to their interests, they are more likely to engage with the content, thereby increasing the chances of conversion.
Moreover, email personalization fosters a sense of connection between the brand and the consumer. In a world where consumers are bombarded with information and advertisements, personalized emails cut through the noise and resonate with recipients on a deeper level. This emotional connection can lead to long-term relationships and repeat business.
Techniques for Effective Email Personalization
To maximize the effectiveness of email personalization, businesses must employ various techniques. These may include:
- Segmentation: Dividing the email list into specific groups based on demographics, purchasing behavior, or engagement levels allows marketers to tailor content to different audiences.
- Dynamic Content: Utilizing dynamic content enables marketers to change elements within the email based on real-time data, ensuring that each recipient receives the most relevant information.
- Behavioral Trigger Emails: Sending automated emails based on user actions, such as abandoned cart reminders or follow-up messages after a purchase, can significantly enhance personalization efforts.
- By using these strategies, companies can craft more captivating and effective email marketing campaigns that truly connect with their audience.
To elevate these personalization methods further, utilizing AI-driven tools can be revolutionary. These sophisticated platforms employ machine learning to assess customer behaviors, forecast preferences, and automatically deliver highly relevant content. Options like Mailchimp, ActiveCampaign, and GoHighLevel provide powerful AI features for segmentation, personalized dynamic content, and behavior-based triggers.
Excited to discover how AI can enhance your email marketing? Take a look at some of these tools and witness the significant impact they can have!
Fundamentals of Machine Learning in Email Personalization
Machine learning is a subset of artificial intelligence that focuses on developing algorithms capable of learning from data and making predictions or decisions without being explicitly programmed. In the context of email personalization, machine learning empowers marketers to analyze vast amounts of customer data, identify patterns, and create personalized experiences that cater to individual preferences.
The integration of machine learning into email marketing is revolutionary. Not only does it streamline the process of data analysis, but it also enhances decision-making capabilities, making it easier for marketers to deliver highly relevant content to their audience.
How Machine Learning Works
At its core, machine learning involves training algorithms to recognize patterns in data. For email personalization, this means feeding the algorithm historical customer data, such as past purchases, email interactions, and browsing behavior. Over time, the algorithm learns to identify trends and correlations, allowing it to predict future behavior and preferences.
For example, if a customer frequently opens emails related to outdoor gear and rarely engages with fashion-related content, the machine learning algorithm will learn to prioritize sending outdoor gear promotions to that particular customer. This capability allows for a highly targeted approach to email marketing.
Types of Machine Learning Models
There are several types of machine learning models that can be employed for email personalization:
- Supervised Learning: In supervised learning, the algorithm is trained on labeled data, meaning that it learns from examples with known outcomes. This model can be used to predict future customer behavior based on historical interactions.
- Unsupervised Learning: Unlike supervised learning, unsupervised learning works with unlabeled data. It identifies hidden patterns and groupings within the data set, making it useful for segmentation and clustering customers based on shared characteristics.
- Reinforcement Learning: This type of learning involves training algorithms through trial and error. The algorithm learns to make decisions by receiving feedback based on its actions, which can be beneficial for optimizing email timing and content delivery.
Understanding these models enables marketers to choose the right approach based on their goals and available data.
Challenges in Implementing Machine Learning for Email Personalization
While the potential benefits of implementing machine learning for email personalization are significant, there are also challenges to consider. These may include:
- Data Quality: Machine learning algorithms rely heavily on high-quality data. Inaccurate or incomplete data can lead to misleading insights and ineffective personalization efforts.
- Complexity: Developing and deploying machine learning models requires technical expertise. Many businesses may struggle with the complexity of implementing these systems effectively.
- Privacy Concerns: As customer data collection becomes more prevalent, businesses must navigate privacy issues and ensure compliance with regulations such as GDPR and CCPA. Balancing personalization with ethical considerations is crucial for maintaining consumer trust.
Despite these challenges, the advantages of utilizing machine learning for email personalization far outweigh the drawbacks, making it a worthwhile investment for businesses seeking to enhance their marketing strategies.
Implementing Machine Learning for Email Personalization
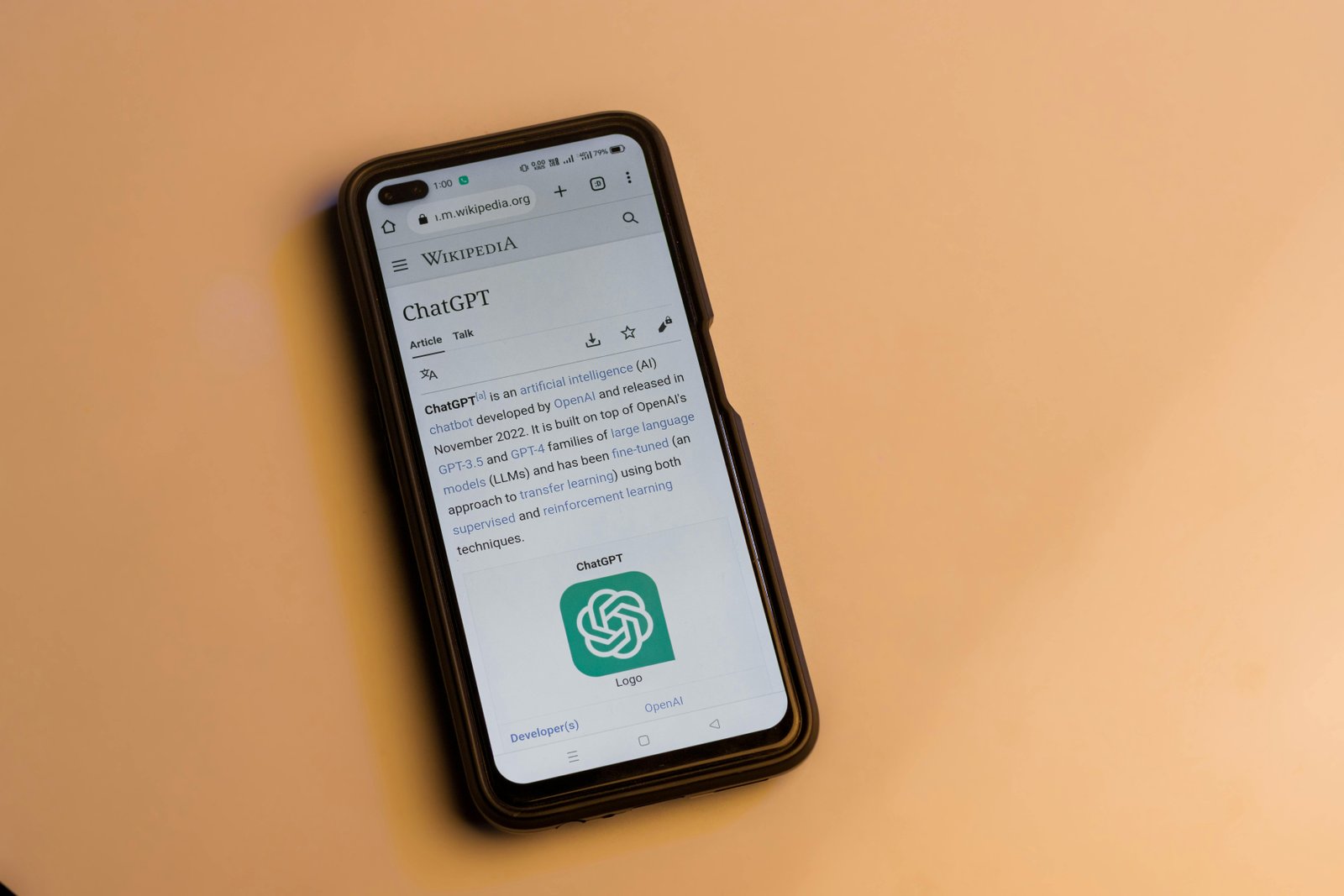
To effectively adopt machine learning for email personalization, companies should take a methodical approach. This includes gathering and examining customer information, developing predictive models for tailored content, and regularly testing and fine-tuning campaigns to enhance their performance.
Gathering and Analyzing Customer Information
The key to successful email personalization is a thorough understanding of the target audience. Companies should collect detailed customer information, encompassing demographics, purchase history, website interactions, and engagement statistics. This data is crucial for training machine learning algorithms.
Here are several methods businesses can use to gather data:
- Website Tracking: Using tracking tools on your site helps you observe user activities, such as visited pages, time spent, and products viewed. This insight reveals valuable information about customer interests.
- Surveys and Feedback: Asking customers for feedback through surveys helps gather qualitative insights about their preferences and expectations.
- CRM Systems: Utilizing customer relationship management systems allows businesses to aggregate data and develop a comprehensive view of customer interactions across various channels.
Once the data is collected, it needs to be cleaned and analyzed to ensure its accuracy and relevance. This step is critical for supplying dependable information to machine learning models.
Creating Predictive Models for Customized Content
With a well-rounded dataset ready, businesses can create predictive models that guide their personalized content strategies. This involves choosing the right machine learning algorithms and establishing key performance indicators (KPIs).
Feature engineering plays an essential role in this phase, as it focuses on selecting and modifying data features (variables) to enhance model performance. For instance, instead of simply relying on raw purchase data, companies could classify purchases by product category, seasonality, or average order value.
After building and training the model, it’s crucial to assess its effectiveness using validation datasets. Evaluating how accurately the model predicts customer behavior enables necessary adjustments before its launch.
A/B Testing and Optimization Strategies
Once machine learning models are operational, it’s crucial to continuously test and refine personalization strategies. A/B testing is an excellent method that allows businesses to assess different versions of an email campaign to identify which one works better.
In the A/B testing process, marketers can analyze various elements such as subject lines, content formats, call-to-action buttons, and the timing of emails. By monitoring important metrics like open rates, click-through rates, and conversion rates, companies can gather valuable insights to drive improvements.
Additionally, utilizing optimization strategies can enhance the success of campaigns even further. This could include adjusting email frequency, experimenting with different content styles (such as videos or infographics), or honing in on segmentation tactics based on new trends.
By dedicating themselves to continuous testing and refinement, businesses can elevate their personalization efforts, resulting in improved customer engagement and a higher return on investment (ROI).
Future Trends in Email Personalization with Machine Learning
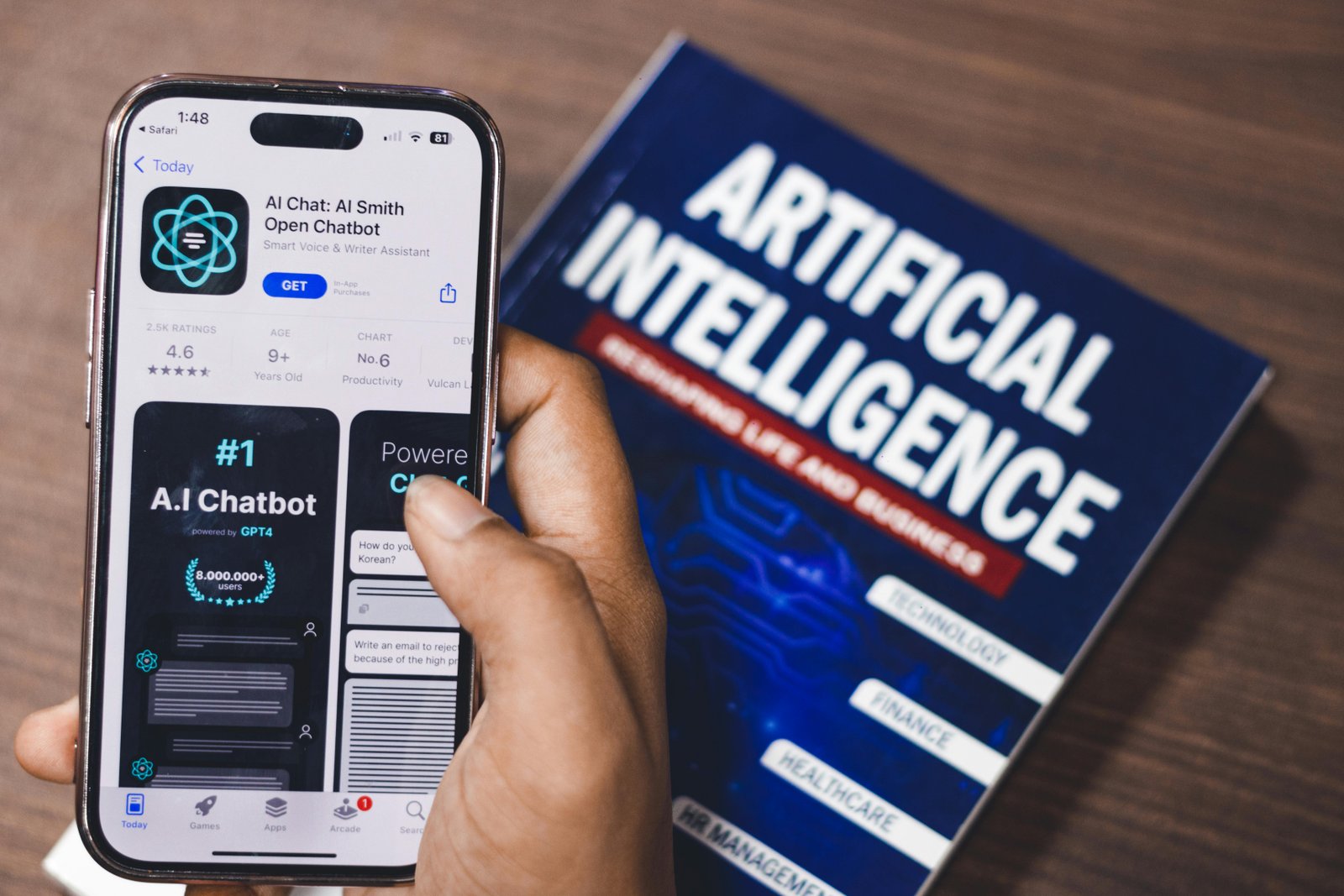
As technology continues to evolve, so too do the possibilities for email personalization. The future holds exciting innovations that promise to enhance the effectiveness of machine learning in email marketing.
Advances in Natural Language Processing (NLP)
Natural language processing (NLP) is a branch of artificial intelligence that focuses on enabling machines to understand and interpret human language. NLP advancements hold immense potential for email personalization, particularly in terms of content creation and sentiment analysis.
By leveraging NLP, businesses can analyze customer responses and sentiment toward previous campaigns, allowing them to tailor future content accordingly. Additionally, NLP can facilitate the automatic generation of personalized email copy, adapting language to align with individual recipient preferences.
Furthermore, NLP can enhance the ability to segment customer lists by analyzing the language used in previous communications. By identifying common themes and topics of interest, marketers can create hyper-targeted campaigns that resonate deeply with their audience.
The Role of Artificial Intelligence in Personalization
Artificial intelligence (AI) plays a crucial role in enhancing email personalization beyond traditional machine learning capabilities. AI algorithms can analyze extensive datasets at unprecedented speeds, uncovering insights that would be difficult for humans to detect.
One significant trend is the use of AI-driven predictive analytics, which allows businesses to anticipate customer behavior and preferences more accurately. For instance, AI can identify the best times to send emails based on individual recipient habits, improving open and engagement rates.
Moreover, AI can automate various aspects of email marketing, including list segmentation, content recommendation, and audience targeting. This automation email frees up valuable time for marketers, enabling them to focus on creative strategy and campaign development.
Ethical Considerations and Data Privacy Issues
As businesses increasingly rely on data to drive email personalization, ethical considerations and data privacy issues come to the forefront. Striking a balance between personalization and consumer privacy is paramount to maintaining trust and credibility.
Organizations must prioritize transparency in how customer data is collected, stored, and utilized. Providing clear opt-in policies and allowing customers to control their data preferences can foster an environment of trust.
Compliance with regulations such as GDPR and CCPA is also essential. These regulations mandate strict guidelines regarding data handling and require businesses to obtain consent from users before collecting personal information.
In addition, ethical concerns surrounding AI and machine learning must be addressed. Companies should ensure that their algorithms are free from bias and discrimination, promoting inclusivity in their personalization efforts.
By taking a proactive approach to ethics and data privacy, businesses can cultivate strong relationships with their customers while harnessing the power of machine learning for email personalization.
Closing Remarks
In conclusion, leveraging machine learning for email personalization marks a major shift in how businesses strategize their marketing. By understanding the intricacies of email customization and applying machine learning, companies can create meaningful interactions with their audience.
As technology evolves, it’s crucial for organizations to adapt to new trends and consider ethical concerns. Prioritizing quality data, transparency, and customer trust enables businesses to maximize the advantages of machine learning in their email campaigns.
Ultimately, adopting these innovations will improve engagement, boost conversion rates, and strengthen customer relationships. The journey towards excellence in email personalization through machine learning is ongoing, but its rewards are significant.